Forest Canopy Density Mapping Software
ITTO Side Event (1)ITTO Side Event (1) SFM for REDD: from acronyms to implementation in the tropics FOREST CANOPY DENSITY MAPPINGFOREST CANOPY DENSITY MAPPING.
Challenge using Software Calculate the change in the percent leaf cover as this maple tree as it greened up in the spring. This can be done by creating a mask based on selecting a range of shades of green, and then measuring the area of the selected green. Download the images of the leaves below and use in the AnalyzingDigitalImages software.
Use the 'Mask Colors' window. See instructions on how to. To download the image, either right-click or click and hold on the image. A pop-up menu will have the option to 'Save Image As.' , which allows you to save the image on your computer. You may do this to download most images available on the Internet. See the on AnalyzingDigitalImages for guidance.
Is available in the DEW software bundle.

Forest canopy density (FCD), estimated with the FCD Mapper, was correlated with basal area and predominant height (PDH) for 48 field plots, measured in highly variable native eucalypt forest at Toolara, south-eastern Queensland, Australia. The Mapper was produced for the International Tropical Timber Organisation and is available on a CD-ROM. It estimates FCD as an undefined index of canopy density using reflectance characteristics of Landsat Enhanced Thematic Mapper images. The Mapper is a 'semi expert' computer program which uses interactive screens to allow the operator to make decisions concerning the classification of land into bare soil, grass or forest. The results of a FCD classification are therefore dependent on the operator's decisions and were found to be highly sensitive to small changes in settings. A positive, weak (r.sup.2 = 0.36) nonlinear relationship of FCD with basal area was observed, while a strong (r.sup.2 = 0.68) similar relationship was observed between FCD and PDH.
The strong relationship of FCD with PDH suggests that this remote sensing technique has promise for forest inventory, but that a quick and robust method of measuring FCD in the field is still required for ground truthing. Keywords: land classification; forests; forest inventories; assessment; remote sensing; landsat; multispectral imagery; canopy; stand density; Queensland.
Introduction There is a continuing need for information about the spatial distribution and density of forests for purposes such as quantifying trends in land clearing in Australia (Kuhnell et al. 1998), estimating national carbon budgets in Sweden (Eklundh et al.
2003) and plantation management (Baynes 1995). In plantations, field measurements of parameters such as stand height and basal area are time consuming and labour intensive. Native forests are inherently more variable in age, species mix and structure, and although aerial photographs have been widely used for native forest inventory, their use over extensive landscapes such as the Northwest Territories of Canada is logistically difficult and economically impractical (Gerylo et al.
Consequently, the need to map the spatial heterogeneity of forests over a range of scales and for a variety of management purposes has led to the widespread application of satellite remote sensing. In the literature, however, there are few remote sensing tools which can be applied to detect change in forest structure (Franklin et al.
In Canada, older vegetation maps, derived from images with pixel sizes ranging from 8 km to 1 km, did not provide sufficiently detailed information because landcover varies over short distances (Cihlar et al. More recent investigations have therefore correlated digital data provided by the Landsat Enhanced Thematic Mapper (ETM) (with a pixel size of 30 m) with forest parameters such as leaf area index (LAI) (Baynes and Dunn 1997; Fernandes et al. 2003; Seed and King 2003). LAI is defined as half the all-sided green leaf area per unit ground surface area projected on the horizontal datum, and is a key parameter in canopy process models (McNaughton and Jarvis 1983). The process of correlating satellite digital data with LAI or other forest parameters requires access to Geographic Information System (GIS) software, an understanding of the theory of remote sensing, and expertise in bio-physical modelling.
Without specialised training, few foresters would have the expertise to estimate LAI using some recently-published algorithms involving shadow fraction and soil-adjusted vegetation indices (Seed and King 2003; Hall et al. Therefore, the FCD (forest canopy density) Mapper has been designed (JOFCA 2003) to provide foresters with a GIS that can be used by semi-experts to process satellite images into maps of forest density. FCD Mapper The FCD Mapper (Mapper) is a computer software package compatible with Microsoft Windows-based personal computers. The Mapper uses the reflectance characteristics of Landsat ETM bands 1-7 with vegetation and bare soil to produce a forest canopy density map.
Processing of the ETM images is done with a 'semi-expert' system wherein the operator makes key decisions concerning the classification of images into bare soil, grass, forest, etc. The end result is a new image, an FCD map, which shows the forest canopy density for each pixel as a percentage of canopy density, from 0% to 100% (JOFCA 2003). As the FCD is not precisely defined in the user guide and is not directly correlated with any parameter of canopy density, it should be considered as an undefined index of canopy or forest density.
The Mapper was produced by the Japan Overseas Forestry Consultants Association (JOFCA) for the International Tropical Timber Organisation (ITTO). The latest version, version 2, was revised as part of ITTO project PD 13/97 Rev.
1 (F) in 2003. It is available from ITTO as a CD-ROM. Objective The user manual (JOFCA 2003) describes version 2 of the Mapper as having achieved a 90% success rate in classifying the canopy density of tropical (evergreen) and monsoon (deciduous) forest.
However, this version had not been tested in wet to dry eucalypt forest in a situation where seasonal swamps and eucalypt woodland are easily confused with grassland or bare ground. The objective of this study was therefore to assess the correlation of FCD with stand height and basal area in eucalypt forests of variable stocking, age, stand structure and species. Background to the operation of the FCD Mapper The theory and operation of the Mapper are described fully in the FCD Mapper User's Guide Ver.
1.1 (JOFCA 1999) and the FCD Mapper User Guide Ver. 2 (JOFCA 2003). It was originally developed as a tool to assess the regrowth of a forest canopy in logged tropical forest. In these forests, a 'type 1' situation is recognised (Rikimaru et al. 1999) as land with a sparse canopy, little understorey vegetation and bare soil visible from space.
A 'type 2' situation exists where canopy density may still be low but understorey vegetation forms a complete ground cover. The Mapper aims to discriminate between type1 and type 2 vegetative cover and then assign an index of canopy density to land with a type 2 cover. The Mapper uses the reflectance characteristics of Landsat ETM bands 1-7 as its data source (Table 1). Briefly, the FCD of a study area is computed from Landsat ETM data, using four main indices: 1. A Vegetation Index (VI), selected from the NDVI (Normalised Difference Vegetation Index), the AVI (Advanced Vegetation Index), or the ANVI (Advanced Normalised Vegetation Index), 2. A Bare Soil Index (BI), 3.
A Shadow Index (SI), and 4. A Thermal Index (TI). These four indices are calculated as new images from the raw ETM bands 1-6 with the thermal band designated as band 7, i.e.
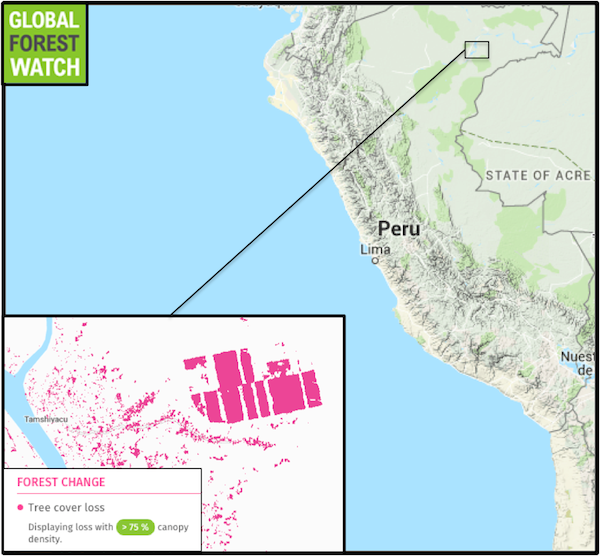
From these indices, the program then calculates a vegetation density (VD), which includes grassland and forest but excludes bare soil. Grassland is then separated from forest using a scaled shadow index (SSI). Finally, a forest canopy density is calculated for each pixel of forested land. The relevant algorithms are:. NDVI = (B4 - B3) / (B4 + B3). AVI = (B4 x (256 - B3) x (B4 - B3) + 1)1/3, (B4 - B3) 0. ANVI, synthesised from NDVI and AVI by principal component analysis.
BI = ((B5 + B3) - (B1 + B4)) / ((B5 + B3) + (B1 + B4)) x 100 + 100. TI = calibrated value of B7. SI = ((256 - B1) x (256 - B2) x (256 - B3)).sup.1/3. VD, calculated from the first principal component of VI and BI. SSI = calibrated shadow index for forested land.
FCD = (VD x SSI + 1).sup.1/2. The program calculates these indices and integrates them into an FCD (as an index from 0 to 100) for each pixel of the final FCD image. The underlying principle for each of the four main indices is that the VI has a negative relationship with the quantity of vegetation, i.e. It decreases from bare soil to grassland to forest. The BI increases as the bare soil increases with increasing site aridity and consequent exposure of the soil.
The high reciprocity of bare soil status and vegetation status is combined using the VI and the BI to assess land as a continuum ranging from dense forest to exposed soil. The SI increases as forest density increases, and this index is used to separate grassland from forest. The TI is less inside the canopy of a forest due to blocking and absorption of the sun's rays and because of the cooling effect of evaporation from leaves.
The TI is therefore used to further differentiate bare soil from grassland and forest. As each index is computed, the operator is required to visually classify the study area into mutually exclusive categories such as bare soil or vegetation. For each classification, the computer screen shows a histogram of the digital reflectance of the image and the operator moves the cursor bar to set threshold levels of the index (Fig.
This operation presupposes that the operator has some knowledge of the vegetative cover of the area and that classification errors will be picked up in subsequent field checking. FCD statistics for particular areas of the image can be calculated using a mask file to exclude pixels outside the area of interest and the FCD map can be exported as a bitmap. Study site and data Location The study site was situated between Toolara Forest (north) and the town of Noosa Heads (south) in subtropical south-eastern Queensland.
The long-term average rainfall at the Toolara Forest Station (26degrees05'S 152degrees50'E) is 1275 mm and, as is typical for coastal subtropical regions, more than two-thirds of this falls in the period October-March. Mean daily maximum and minimum temperatures are 29.6 and 20.2degreesC, respectively, in January, and 21.6 and 6.3degreesC, respectively, in July. FIGURE 1 OMITTED Soils The terrain is relatively flat with an altitude above sea level of less than 150 m, except for the Cooran Tableland which has a maximum elevation of 483 m over an area of about 5000 ha. Soils on the hilly and inland areas are derived from a variety of metamorphic, sedimentary and volcanic rocks (Young and Dillewaard 1999), whereas the eastern side of the study area consists of deep coastal sands and shallow podzols subject to periodic inundation (Stace et al. Vegetation For the purposes of remote sensing, vegetation over the study area consists of plantations of pine trees, agricultural grassland and a wide range of native forest. Native vegetation is dominated by mixed stands of Eucalyptus and Corymbia species, with some pure stands of individual species.
The species mix is mainly determined by soil fertility and water availability. The coastal sands particularly are phosphorus deficient, and tree cover, at its poorest level, is reduced to scattered mature trees, principally scribbly gum (E. Signata F.Muell.) with a height of 40 m. Finally, 'wet eucalypt' forest, of which rose gum (E. Grandis W.Hill ex Maiden) is a principal species, is found in association with rainforest in wet gullies and sheltered sites. The rainforest comprises a number of overstorey and understorey species including trees, vines, palms and ferns. In summary, the native vegetation is highly variable and many species exist across the entire geographic range.
Species intermixing rather than pure stands is the rule, but the broad vegetation types described above can be discriminated with suitable aerial photographs. Remotely-sensed data A Landsat 7 ETM (52 x 69 km) image of the study site, taken on 16 September 2000, was obtained for bands 1-7. The image had been radiometrically and geometrically corrected to remove detector-to-detector and band-to-band brightness variations and image distortions. The image had also been resampled to a 25-m grid and aligned to the Gympie 1:100 000 mapsheet using ground control points. As the original ETM thermal image was supplied with a pixel size of 50 m, this image was converted to pixels of 25 m using the EXPAND utility of the IDRISI GIS software.
All images were then exported to the Mapper as TIFF files. From previous experience in this region, September is ideal for the collection of remotely-sensed data used to discriminate between vegetation types. This is because annual grasses have died off through frost or dryness, and contrast clearly with perennial vegetation. The image was cloud free; one area of bushfire smoke which was present was ignored in the subsequent analysis. No rain had fallen in the previous 14 days, and the last rainfall recorded at the Toolara Forest Station was only 1.2 mm. Method FCD Mapper The Landsat image was loaded into the Mapper and processed as per instructions in the user guide. To assist classification of the study area, eight reference positions were chosen, ranging from bare soil to forest with a closed canopy, i.e.
Dry white sand, a recently clear-felled pine plantation, dry red soil, an airport tarmac, a swamp covered with dense green vegetation, poor-quality bloodwood forest, Gympie messmate forest and wet eucalypt forest. Processing was taken to the stage of calculating the initial FCD map. The program permits further processing to adjust the FCD map to forest canopy information derived from ground data points using regression analysis. This was not done, as the aim of the investigation was to assess the performance of the Mapper in estimating stand basal area or predominant height (PDH, the average height of the tallest 50 trees ha-1) over a range of forest types. To assist interpretation of the results of the FCD map, spectral signatures were calculated for ETM bands 1-5 for the swamp, bloodwood, Gympie messmate, blackbutt and wet eucalypt forest. From a field inspection, five positions were chosen across the range of each landcover and the reflectance of a matrix of 2 x 2 pixels was recorded for each position. Reflectance values were obtained from each image using the interactive query function in IDRISI and a mean reflectance of the 20 pixels calculated.
Also, the algorithms used in the Mapper were used to calculate BI, TI and SI for each landcover. Plot selection To check the accuracy of the FCD classification, the PDH and basal area (i.e. m.sup.2 ha.sup.-1 of tree cross sectional area at 1.3 m above ground level) were measured on 48 field plots of 0.1 ha. Twelve plots each were measured in (1) wet eucalypt and rainforest, (2) blackbutt forest, (3) Gympie messmate and spotted gum forest, and (4) poor-quality bloodwood forest.
Plots were not measured in the wallum as the tree cover is highly variable, often with stockings of less than 50 trees ha.sup.-1. Plot positions were located on the FCD image and the coordinates transferred to a Global Positioning System for location in the field. The plots were located in uneven-aged mature forest to minimise growth differences between the time of image capture (2000) and plot measurement (2003).
Results Field plots Mean basal area was 27.8, 22.5, 22.6 and 15.9 m.sup.2 ha.sup.-1 for the wet eucalypt, blackbutt, Gympie messmate and bloodwood forest plots, respectively (Table 2). Mean PDH was 39.6, 40.3, 37.4 and 13.9 m for the same forest types. Mean FCD declined similarly from 61 to 56, 45 and 36 for each landcover, respectively. A weak, non-linear relationship (r.sup.2 = 0.36), best described by a power function, was observed between basal area and FCD (Fig.
A strong non-linear relationship (r.sup.2 = 0.68), also best described by a power function, was observed between PDH and FCD (Fig. FCD image The FCD image classified 35.7% of the 223 852 ha study area as grassland, bare soil and surface water. This included agricultural fields and wallum in which vegetation cover was incomplete.
Surface water had been masked out during processing. The remaining 64.3% of the study area was classified as having an FCD ranging from 1% to 80%. No forest was classified as having an FCD above 80%. As the wet eucalypt reference area has a closed canopy and a high level of shadow, it would be expected that the FCD of this forest type would be close to 100%. The estimates of FCD are therefore lower than expected, at least for the wet eucalypt forest. Provision is made in the program to correlate FCD percentage values with ground control points to re-calibrate the FCD values. This is difficult, however, as the corrected data are input as FCD percentages and no method is offered for measuring FCD in the field.
FIGURE 2 OMITTED FIGURE 3 OMITTED In addition, the swamp reference point (which is covered with dense green vegetation) was classified as having an FCD of 0% - 30%. A field check and aerial photographs showed that this was an overestimate, as there were only isolated trees growing in the swamp. The program was rerun and the classifications altered to classify the swamp as grassland, using a process similar to that described for Figure 1.
The SSI setting was altered to classify more of the image as grassland and less as forest. However, reclassifying swamp to grassland altered the settings of the FCD map (Fig.
4a and 4b) over the entire FCD range. For instance, the first run of the program classified 45% of the study area as FCD = 0, while the second run classified 51.2% as FCD = 0, as expected. However, this also had the effect of reducing the number of pixels with an FCD range of 60-70% from 11 209 to 7799. The Mapper is therefore highly sensitive to the settings used by the operator. The spectral signatures of the forest types and the swamp (Fig. 5, Table 3) are similar.
The swamp has the least vegetative cover and as expected, it shows the highest reflectance in bands 1, 2, 3 and 5 and the lowest reflectance in band 4. This has resulted in a BI slightly higher than the other forest types and a SI which is slightly lower. The TI of the swamp (137) is similar to the TI of dry white sand (140). FIGURE 4 OMITTED FIGURE 5 OMITTED Discussion and conclusion Traditionally, the term 'stand density' has had several meanings for foresters, as it involves the concept of stand crowding or competition between members of the stand, which is in turn determined for a particular age by stand stocking and stand basal area (Baskerville 1962).
Various indices of stand density, usually incorporating stocking, tree height, diameter and canopy measurements are well described in the literature (Smith 1962; Carron 1968; Hocker 1979). However, indirect methods of canopy density estimation using 'fish eye' photographs or instruments such as ceptometers or spherical densiometers are liable to bias and are time consuming to use (Roy 1999).
Hence recent investigations have used LAI, estimated with satellite imagery, as a principal index of forest or canopy density. Intuitively, the algorithms used in the FCD Mapper provide an index of LAI, even though the user guide describes FCD as being 'the degree of forest density, expressed as a percentage' (JOFCA 1999).
Therefore, the challenge is how best to measure and extract image variance that maximises information on LAI of the forest overstorey (Seed and King 2003). The Mapper was successful in explaining a high percentage of the variation between PDH and FCD, but less so with basal area. The weak relationship between FCD and basal area is not unexpected because the plots were chosen over a wide range of stocking, species mix and tree size. Stocking, particularly, was highly variable in all forest types.
However, the high correlation of FCD with PDH indicates that the Mapper discriminates between forest types over a wide range of forest types. Tree height is relatively unaffected by stocking (Lanner 1985; Oliver and Larson 1996). It is possible that in mature stands of variable stocking but complete crown cover, PDH is a more reliable indicator of crown density, when plot sizes are small (0.1 ha), than basal area.
The Mapper is sensitive to changes to the settings which control the classification of land as bare soil, grassland or forest. These classifications are controlled by the operator, and where vegetation types and boundaries are distinct there should be no problem achieving a correct separation of landcover types. However, where the contribution of the understorey becomes significant, such as in wallum heath and swamp, and the spectral signature of the understorey is similar to that of the overstorey, then confusion between vegetation classes may result. In this study the author had a detailed knowledge of the study site, but without this knowledge misclassification of the vegetation classes would be a likely result. Consequently, the settings used for projects undertaken with the Mappper should be recorded, particularly if the Mapper is to be used for time series analysis.
The study area in this project was reasonably large and encompassed a wide variety of vegetation types. Reducing the size and variation of the study area with mask files may assist the operator to discriminate between the vegetation types.
In conclusion, it would be most useful to have a robust field method of correlating initial FCD percentages with a field measurement of forest or canopy density. This would enable foresters to correlate FCD, which at present is just an index of reflectance, to a parameter which can be measured in the field. This could considerably enhance the usefulness of the Mapper to field staff. Revised manuscript received 8 June 2004 References Baskerville, G.L. (1962) Production in Forests.
Density Mapping Software
Forest Research Branch, Department of Forestry, Canada. (1995) Site index prediction in plantations using LANDSAT Thematic Mapper digital data. And Greenwood, C. Applications of New Technologies in Forestry.
Proceedings of Institute of Foresters of Australia 16th Biennial Conference. Ballarat, Australia, pp. And Dunn, G.M. (1997) Estimating foliage surface area index in 8-year-old stands of Pinus elliottii var. Elliotii x Pinus caribaea var. Hondurensis of variable quality. Canadian Journal of Forest Research 27, 1367-1375.
(1968) An Outline of Forest Mensuration. Australian National University Press, Canberra. Cihlar, J., Guindon, B., Beaubien, J., Latifovic, R., Peddle, D., Wulder, M., Fernandes, R. (2003) From need to product: a methodology for completing a land cover map of Canada with Landsat data. Canadian Journal of Remote Sensing 29, 171-186. (1971) The Language of Botany. Society for Growing Australian Plants, Sydney, 208 pp.
Eklundh, L., Hall, K., Eriksson, J., Ardo, J. And Pilesjo, P. (2003) Investigating the use of Landsat Thematic Mapper data for estimation of forest leaf area index in southern Sweden. Canadian Journal of Remote Sensing 29, 349-362. Fernandes, R., Butson, C., Leblanc, S. And Latifovic, R. (2003) Landsat5 TM and Landsat 7 ETM+ based accuracy assessment of leaf area index products for Canada derived from SPOT-4 vegetation data.
Canadian Journal of Remote Sensing 29, 241-258. Franklin, S.E., Lavigne, M.B., Wulder, M.A. And McCaffrey, T.M.
(2002) Large-area forest structure change detection: an example. Canadian Journal of Remote Sensing 28, 588-592. Gerylo, G.R., Hall, R.J., Franklin, S.E.
And Smith, L. (2002) Empirical relations between Landsat TM spectral response and forest stands near Fort Simpson, Northwest Territories, Canada. Canadian Journal of Remote Sensing 28, 68-79. Hall, R.J., Davidson, D.P.
And Peddle, D.R. (2003) Ground and remote estimation of leaf area index in Rocky Mountain forest stands, Kananaskis, Alberta. Canadian Journal of Remote Sensing 29, 411-427. (1979) Introduction to Forest Biology. John Wiley and Sons, New York. (1999) FCD-Mapper Ver. User's Guide.
International Tropical Timber Organisation and Japan Overseas Forestry Consultants Association. Yokohama, Japan.
(2003) FCD-Mapper Ver. International Tropical Timber Organisation and Japan Overseas Forestry Consultants Association.
Yokohama, Japan. Kuhnell, C.A., Goulevitch, B.M., Danaher, T.J. And Harris, D.P. (1988) Mapping woody vegetation cover over the state of Queensland using Landsat TM imagery. In: Proceedings of the 9th Australasian Remote Sensing and Photogrammetry Conference. Sydney, Australia. CD, Causal Productions, and slats/report.html (cited 20 February 2004) 22 pp.
(1985) On the insensitivity of height growth to spacing. Forest Ecology and Management 13, 143-148. McNaughton, K.G. And Jarvis, P.G. (1983) Predicting effects of vegetation changes on transpiration and evaporation.
In: Kozlowski, T.T. (ed.) Water Deficits and Plant Growth Vol. Academic Press, London, pp. And Larson, B.C. (1996) Forest Stand Dynamics.
Update edition. John Wiley & Sons, New York. Rikimaru, A., Miyatake, S. And Dugan, P.
(1999) Sky is the limit for forest management tool. Tropical Forest Update 9, 6-8. (1999) Space remote sensing for forest management. In: FCD-Mapper Ver. User's Guide. International Tropical Timber Organisation and Japan Overseas Forestry Consultants Association.
Yokohama, Japan, pp. And King, D.J. (2003) Shadow brightness and shadow fraction relations with effective leaf area index: importance of canopy closure and view angle in mixed wood boreal forest. Canadian Journal of Remote Sensing 29, 324-325.
(1962) The Practice of Silviculture. John Wiley and Sons, New York.
Stace, H.C.T., Hubble, G.D., Brewer, R., Northcote, K.H., Sleeman, J.R., Mulcahy, M.J. And Hallsworth, E.G. (1968) A Handbook of Australian Soils.
Rellim Technical Publications, Glenside, South Australia. Young, P.A.R. And Dillewaard, H.A. (1999) Southeast Queensland. In: Sattler, P.
And Williams, R. (eds) The Conservation Status of Queensland's Bioregional Ecosystems. Environmental Protection Agency, Queensland, pp.
Jack Baynes Department of Natural and Rural Systems, University of Queensland, Gatton, Queensland 4343, Australia; and Gympie Training Centre, Gympie, Queensland 4570, Australia Email: jack.baynes@dpi.qld.gov.au Table 1. Wavelength and characteristics of the seven Landsat ETM bands Band Bandwidth (micro m) Characteristic 1 0.450-0.515 Visible blue 2 0.525-0.605 Visible green 3 0.630-0.690 Visible red 4 0.76-0.900 Near infrared 5 1.550-1.750 Middle infrared 6 10.40-12.50 Thermal 7 2.09-2.35 Middle infrared Table 2. Mean basal area, predominant height (PDH) and forest canopy density (FCD) for 12 plots each in wet eucalypt, blackbutt, Gympie messmate and bloodwood forest at Toolara, south-eastern Queensland Basal area PDH FCD Forest type (m.sup.2ha.sup.-1) (m) (%) Wet eucalypt 27.8 39.6 61 Blackbutt 22.5 40.3 56 Gympie messmate 22.6 37.4 45 Bloodwood 15.9 13.9 36 Table 3. The indices BI, TI and SI calculated for dry white sand, a swamp, bloodwood forest, Gympie messmate forest, blackbutt forest and wet eucalypt forest at Toolara, south-eastern Queensland Bare Soil Thermal Shadow Surface Index Index Index Dry white sand 100 140 0 Swamp 94 137 188 Bloodwood 88 80 200 Gympie messmate 83 81 214 Blackbutt 78 96 211 Wet eucalypt 64 30 227.